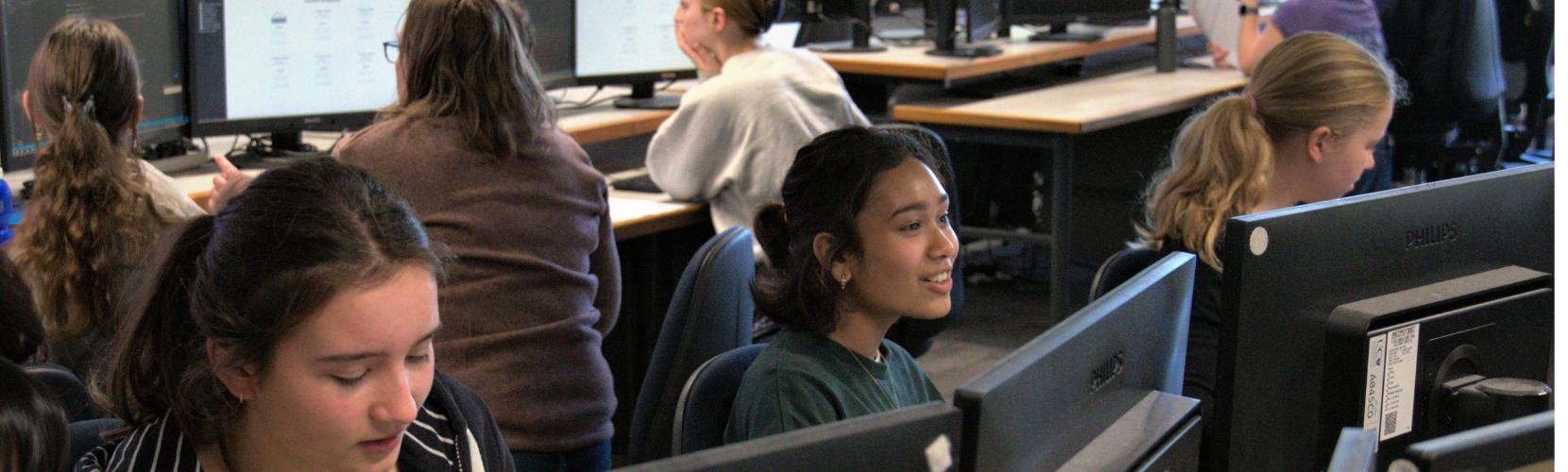
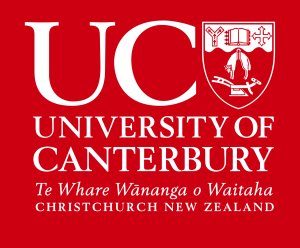
Computer Science and Software Engineering department
Te Tari Pūhanga Pūmanawa Rorohiko
Computer Science and Software Engineering, designing computer systems to help people work efficiently and effectively.
When people think of Computer Science and Software Engineering they often think of programming, but there are many more aspects to it. Learn more and check out UC's department of Computer Science and Software Engineering.
Computer Science and Software Engineering are about designing computer systems to help people do their work efficiently and effectively.
When people think of Computer Science and Software Engineering they often simply think of programming, but there are many more aspects to it. Our department is in the forefront of
- AI & Machine Learning
- Deep Learning
- Data Science
- App Development
- Mobile Systems
- Internet of Things (IoT),
- Autonomous Robots and Drones (AI software)
- Cyber Security
- Medical Imaging
- Intelligent Tutor Systems
- Human-Computer Interfaces
- Software Engineering (Agile, Project Management,…)
- Computer Vision
- Computer Graphics
- Databases
- Computer Science Education
- Networking (Mobile, Ad-Hoc…)
- Sensor Networks
- Computer Music
- Precision Agriculture
The Department of Computer Science and Software Engineering (CSSE) at the University of Canterbury has a strong international reputation and courses that are reviewed regularly against international standards. Our graduates are in strong demand. Staff are active researchers, with collectively one of the best records of research publications in the Pacific region.